This blog is part of a series to help organizations of any size optimize their security. Our experts provide insights and recommendations based on common security use cases, customer questions, and security software developer needs.
Reach. Exploit. Repeat. The expanding number of devices and control points in today’s remote, hybrid, distributed, and often global IT environments are fertile ground for cyberattacks and threat actors.
Attack surface expansion is the forefront of opportunity for cybercriminals, but the right tools and teams can harden security postures and help detect, protect, and respond to threats before they cause maximum harm.
What are the best options to mitigate and manage attack surface expansion?
The two main options organizations have are to increase their staff or to implement AI and machine learning.
Hiring lots of people for cybersecurity vigilance isn’t realistic for most businesses. Not only is it an expensive and time-consuming endeavor, but this tactic must account for shortages in the talent market of people with the specific skills that many organizations need depending on their unique environments.
That leaves implementing smart technology in the forms of artificial intelligence (AI) like machine learning as the better and more effective option to detect and respond to threats. Automation and reliable analytics help round out the technology needed to protect attack surface expansion.
What is the role of AI in cybersecurity?
AI can help IT and security teams process insights rapidly to find threats faster, reducing response times. It can analyze billions of data points to recognize suspicious IP addresses, malicious files, or unusual activity in a technology ecosystem. And AI can do this quickly and continuously, cutting down on previously manual and time-consuming tasks and automating some of the critical decisions that can make all the difference in avoiding impairment from a cyberattack.
Sophisticated algorithms can help train AI-based systems to detect threats such as malware and ransomware with predictive intelligence and natural language processing. As insights are gained from a technology ecosystem, AI-based systems learn to predict breach risk and where organizations are most likely to be compromised to allow for planned responses to vulnerabilities and security gaps.
How can AI help with endpoint protection?
Because endpoints are the gateway to access, they are the greatest targets of malicious and threat actors. A Zero Trust framework helps harden an organization’s security posture by constantly authenticating users and their access privileges from both inside and outside an organization’s network.
AI-based systems support the Zero Trust framework when they are trained to mitigate risks from authenticated access by learning to recognize baselines of behavior for different user personas. AI can learn to take action when outlier behaviors or access occur, such as sending a notification to staff or reverting devices to a safe state.
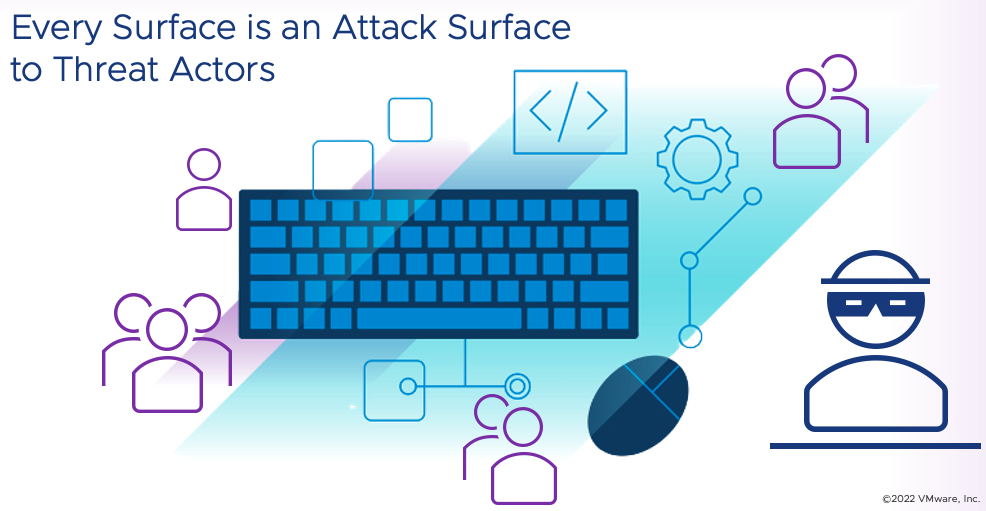
Are there any downsides to using AI for cybersecurity?
The biggest hurdle in using AI-based systems for cybersecurity is that these systems need to be trained, and the best way to train AI for machine learning is to use very large datasets. Datasets are used in many real-world, everyday circumstances. For example, they help applications recommend songs or movies we may like, predict healthcare outcomes, and match similarly skilled video game players.
Huge volumes of data can be very expensive, and there’s no guarantee that even cybersecurity-trained AI won’t render incorrect results or false negatives or positives. Another risk when using datasets to train AI to look for the most current versions of malicious code or anomalies is that some datasets may be inaccurate or from unreliable sources. Some datasets are time-sensitive and may require updates.
Common sources for AI and machine learning datasets include Google’s Datasets Search Engine, Kaggle Datasets, and datasets maintained by big tech companies. Organizations can vet dataset resources by finding other users who have similar organizations and use cases or by using an experienced partner or vendor to purchase datasets.
What is adversarial AI?
Unfortunately, as cybersecurity efforts become more advanced, so do cybercriminals. Adversarial AI is machine learning that causes cybersecurity-trained AI to misinterpret inputs and allow attackers and threat actors to gain access to an organization’s network.
Adversarial AI “fools” cybersecurity models with deceptive data through the development of malicious machine learning algorithms. It exploits an AI-based system by manipulating or deceiving learned behavior so that the new malicious algorithms can get traction within that environment.
Another form of adversarial AI is adversity caused by incidental circumstances, such as data corruption.
How can AI-enabled cybersecurity become more secure against adversarial AI?
The emerging field of adversarial robustness aims to help AI-based systems become more secure and resilient. The focus of adversarial robustness is to continuously look for vulnerabilities within an AI-based system and remedy them so that the system is less likely to be deceived by malicious algorithms.
Some vulnerabilities lie in datasets that include or exclude certain parameters that make them more vulnerable to data poisoning. Neutralizing these types of threats may include teaching systems to use fake dataset parameters below a certain threshold, allowing for true results without causing a vulnerability. Another consideration is to distribute the weight of certain datasets or data points to minimize loss in the event of an adversarial attack.
One more tactic is to allow machine learning of a dataset without labels. This allows the system to recognize data points with similarities or contrasts to each other rather than rely solely on labels to differentiate data points. This creates a more robust system that has learned how to match data points without labels, making it less vulnerable to corrupt or malicious data points.
Get started on slowing the risks of attack surface expansion
If you’re not sure about your security posture or the level of vulnerability in your organization’s IT environment, a security assessment can help you develop a clear view of your current state and possible remediations needed. Visit the Professional Services for Security resources section for overviews on the different types of assessments available.
Learn more about security assessments and security operations on our Professional Services for Security pages or contact us at [email protected].
For more support, read the other blogs in this series:
- The Security Toolbox: Building a SOC on a Budget – Learn about the common tools and methodologies for a security operations center
- The Security Toolbox: Meet Cybersecurity Mesh Architecture – Discover the promise of better security through the concept of CSMA