by Kristopher Groh, Director, Product Management and Shubha Rajan, Staff Technical Partner Manager, VMware vSAN
The rise of AI is affecting operations all over the enterprise. Edge infrastructure, including storage, is facing challenges around storage and data management for artificial intelligence (AI) and machine learning (ML) workloads. These challenges include diverse deployment environments, complex storage/data management solutions, high cost, and a lack of skills that can delay adoption. To help address this complexity, VMware is bringing simplicity to the development of edge-native AI/ML applications. A secure, robust, and predictable application delivery platform that includes VMware Edge Compute Stack and VMware vSAN provides crucial support and helps organizations prepare for an AI future.
In this article, we first observe the trends in AI-infused workloads at the edge that affect infrastructure. Then we describe how VMware Edge Compute Stack with VMware vSAN solves many aspects of Edge AI challenges. We also discuss how data management is an area where VMware strives to achieve further simplicity, and list notable VMware long-term initiatives.
AI business opportunities require quick infrastructure decisions
We live in a data-rich world: sensors and cameras are pervasively deployed. Machines used in manufacturing can self-monitor, report issues, and even self-heal.
AI at the edge is in its infancy, and is rapidly evolving. There are new opportunities to gain business insights and optimize operations. AI and ML models can help organizations take advantage of these opportunities, benefit customers, and derive business value. Businesses need to plan and execute to accommodate changing application requirements at the edge. VMware’s software-defined infrastructure approach adds the ability to re-architect without re-visiting physical infrastructure.
AI is already known to have improved innovation, sustainability and customer and employee experience in a variety of applications. Early adopters report 30-35% improvement of business outcomes with the rollout of AI solutions. (Source: IDC, Infrastructure for AI at the Edge).
Edge deployments with machine learning are expected to increase 10X, from 5% in 2022 to 50% in 2026. (Source: Gartner®, Building an Edge Computing Strategy, Thomas Bittman, 26 May 2023)
IDC reports a large and growing edge spend, estimated at $317B in 2026. AI at the edge is a large component of this, widely expected to be at the cusp of explosive growth.
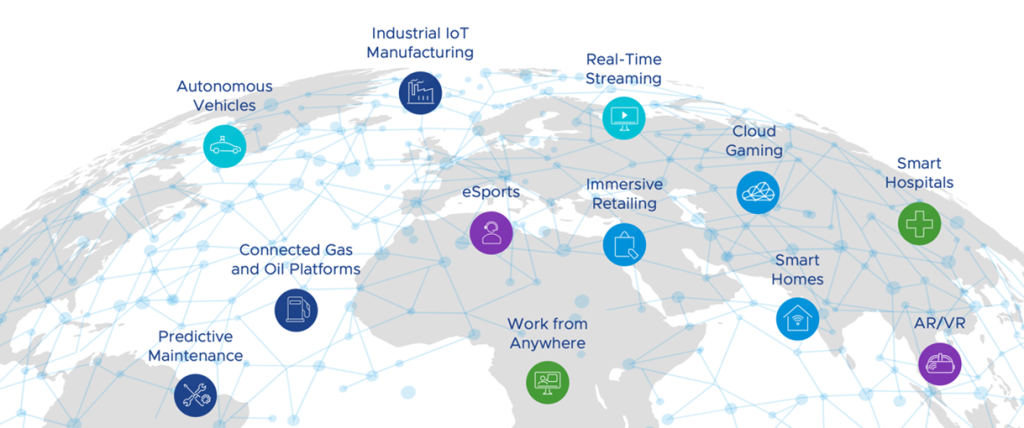
Infrastructure challenges in deploying AI at the edge
Increasingly, AI applications are being deployed in diverse environments outside of-public cloud. Example applications include radiological image processing and automated diagnosis in healthcare.
The diagram below from IDC shows how AI stacks are complex and include storage at every layer.
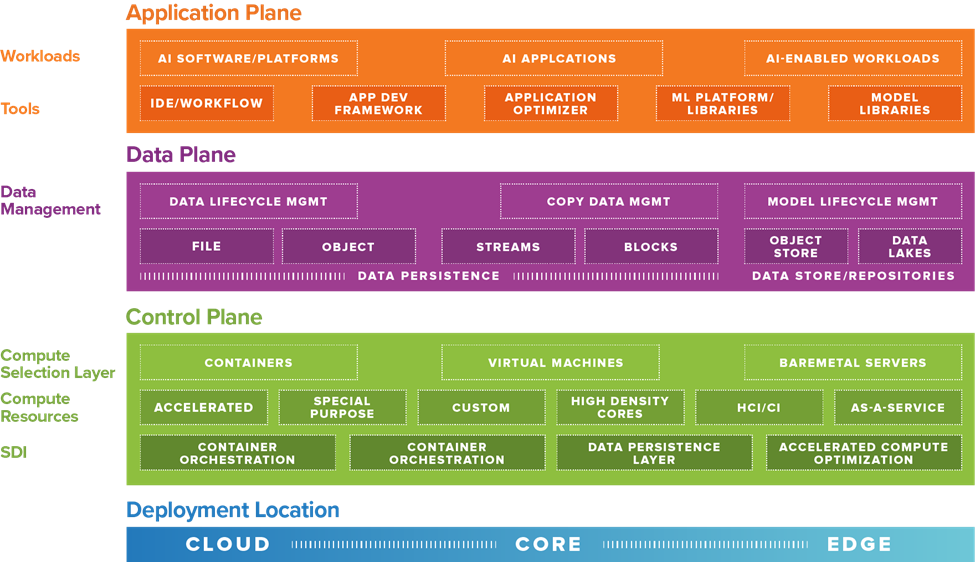
1. Deployment environments are diverse
ML training for an enterprise’s most effective models will be carried out in the cloud, with data gathered from each edge location in real time. Models running at the edge will be continually updated. Enterprises not only must deliver applications to diverse cloud and edge clusters; they also must orchestrate workloads across the clusters and manage the flow of data back and forth. All this calls for a rich and flexible stack.
AI model lifecycle platform workloads are also important. These include tools and technologies used by data scientists and ML developers—including data labeling, AI-built machine learning operations, and trustworthy AI—from experimentation to production deployments.
2. At the edge, storage and data management is expensive
There is a high level of uniformity to the platforms deployed in on-premises and public clouds. This leads to standard tools, automation, and processes for data and storage management. But edge applications greatly expand the range of platforms and deployment environments.
Storage should be available locally. Durability and availability requirements must be satisfied. Data access options range from high-performance block storage to flexible shared files and cost-effective large-scale object storage. In addition, management requirements include backup, snapshots, rollback, and policy-based replication.
Transferring data between edge locations and datacenters is expensive and the latency burden needs to be assessed against the use cases and applications at the edge. The infrastructure stack at the edge should optimize network communications for both the cost-effectiveness to businesses and value to customers.
3. Lack of skills leads to an AI adoption gap
According to an AI Infrastructure View Survey conducted by IDC, the lack of proper storage infrastructure is one of the leading causes of AI project failure. 80% of those surveyed were planning to upgrade their storage infrastructure to support AI workloads.
Today, the infrastructure stack used to train AI and to use the ML models for inference is complex and different from existing hardware running and managing a general-purpose datacenter. The skills required to manage AI infrastructure are in short supply, which is one of the main factors in AI adoption. According to IDC, 32% of businesses surveyed said that the lack of AI infrastructure skill sets is an impediment for AI adoption.
Trends in edge workloads
The physical world is becoming automated. Businesses are deploying new solutions where people, things and data connect to the networked digital world. As these solutions grow in sophistication, they increasingly use the techniques of AI/ML.
Deploying AI at the edge further helps in the trend towards true edge-native applications: to a degree, the available intelligence at the edge location enables the edge location to operate autonomously.
Edge is key. But it is only one component of the overall intelligent solution. An enterprise will have numerous edge locations. Training for the models running in these locations will be done centrally, leveraging the data collected in all of them. Models are trained in the cloud or on-premises and deployed to edge locations. Sensor, camera, and other data are input at the edge, used for ML inference, and fed back to the cloud for training.
Storage requirements at the edge will increase to handle the collection of rich media inputs for ML inference, and its temporary retention. This leads to the growth of unstructured data which can be used for analytics and ML.
The next frontier – VMware has your back!
Application delivery must be coordinated across cloud and edge clusters
Successfully running an edge-native AI/ML application is an architecturally complex endeavor. VMware has been at the forefront of designing for an intelligent edge, and aggressively worked on achieving simplicity in the solutions that our customers can use.
AI and ML applications are among the newest and most innovative ones being developed. VMware provides crucial developer support with a secure, robust, and predictable application delivery platform.
Uniform management of edge devices and workloads
Across use cases, edge deployments exhibit a diversity of scale and size, and are composed of a wide variety of devices and workloads. VMware vSphere and VMware Tanzu Kubernetes Grid support these deployments with uniform and familiar management interfaces and practices. In addition, there is recent, edge-specific product innovation:
- VMware Edge Cloud Orchestrator will streamline orchestration of edge compute, applications, security, and network services. It incorporates Project Keswick, which provides zero-touch, one-button provisioning and management of devices at various edge locations.
- VMware Edge Compute Stack is a purpose-built, integrated virtual machine and container-based stack that enables organizations to modernize and secure edge-native apps at the far edge.
The vSphere with Tanzu platform dramatically simplifies application development and forms a solid underlying foundation for AI and ML application development.
Uniformly managed storage at different scales
vSphere includes a collection of well-integrated, versatile answers to numerous storage challenges:
- vSAN ESA provides underlying storage, with built-in data redundancy and scale.
- It supports multiple form factors: two and three node clusters, or much larger ones.
- Storage replication: currently, the primary use case is for site reliability. In the future, this may be more flexibly used, for instance for data management.
- vSAN Max is the ideal storage to future-proof for AI workloads with incremental and predictable scalability and provide for flexible ratios of compute and storage.
Data management will be key
VMware plans to address data management challenges, including:
- Federated storage: For instance, common volumes can be used to deliver updated ML models to edge locations in real-time, as they are refreshed.
- Data mesh: While ensuring security, access control, and data governance, data can be moved to and from edge locations and the cloud, automatically driven by configured policies.
VMware is planning to enhance vSAN management to support this new data management interface, and it will continue to remain tightly integrated with the VMware stack.
Meet us at VMware Explore Barcelona to learn more
VMware is committed to putting robust end-to-end solutions in the hands of customers by partnering with key players in the ecosystem for Edge AI. Watch for exciting sessions at VMware Explore Barcelona, including:
- Taking a Cloud-Smart Approach to Harness the Power of Generative AI [GEN2154BCN]
- Solution Keynote: Harnessing the Power of Data and Intelligence for Today’s Changing Workplace [EUSK2160BCN]
- Solution Keynote: Everything Everywhere All at Once – Living on the Edge with VMware [CEIK2161BCN]
- Solution Keynote: Accelerate Application Delivery for Continuous Innovation [MAPK2159BCN]
- Introducing vSAN Max: Petabyte-scale Disaggregated Storage [CEIB1024BCN]